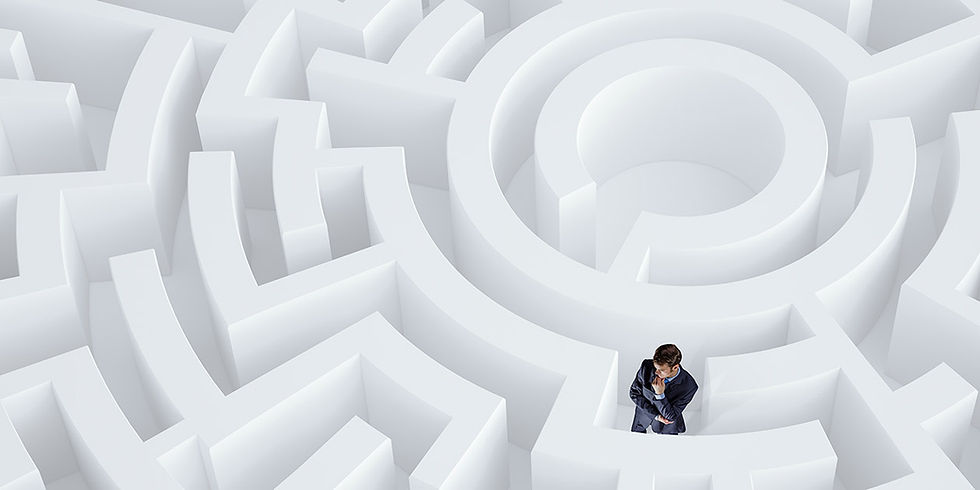
In this era of data-driven decision-making, organizations increasingly turn to AI-enabled analytics to extract valuable insights from vast amounts of data. However, the journey toward successfully implementing these advanced analytics solutions can be fraught with challenges.
One of the primary hurdles faced by companies is ensuring the quality and accessibility of data. This article delves into the intricacies of data quality and accessibility, explores their impact on AI-enabled analytics, and discusses strategies for overcoming these challenges.
Data Silos and Disparate Formats
Many organizations grapple with data silos, where data is scattered across different systems and departments. This makes it tough to consolidate and access data for analysis. Plus, disparate data formats and inconsistent data quality pose additional obstacles.
Different systems may employ diverse data structures, making it difficult to integrate and harmonize the data for analysis. This lack of data integration hampers the ability to gain a comprehensive view of the business. It limits the effectiveness of AI-enabled analytics solutions.
Here’s what you can do:
Establish a robust data governance framework to address this challenge.
This framework should include data integration strategies that enable seamless data consolidation from multiple sources and promote standardization of data formats.
Implementing data integration tools and platforms can streamline transforming and merging data from diverse systems, ensuring accessibility and compatibility for AI-driven analytics.
Inconsistent Data Quality
Data quality is paramount for accurate and reliable insights. However, most organizations need consistent data quality; without missing values, incomplete records, or inaccuracies.
These problems can be attributed to data entry errors, lack of validation processes, or the absence of data quality controls. Data quality is needed to improve the effectiveness of AI-enabled analytics models, preventing skewed results and unreliable predictions.
Here's what you can do:
Invest in data quality management practices.
Implement data profiling techniques to identify data quality issues.
Establish data validation processes.
Deploy data cleansing and enrichment strategies.
Employing data quality tools and technologies can help automate these processes, ensuring that the data used for AI-enabled analytics is accurate, complete, and consistent.
Access to Structured and Unstructured Data
In addition to structured data from databases and spreadsheets, organizations increasingly deal with unstructured data, such as text documents, images, and social media content.
Incorporating unstructured data into AI-enabled analytics poses unique challenges, as traditional analytics techniques may not be suitable for processing and extracting valuable insights.
Here's what you can do:
Leverage advanced technologies like natural language processing (NLP) and computer vision.
Examples of computer vision techniques include:
Object detection
Image segmentation
Image classification
Utilizing generative AI for model training
NLP techniques include:
Sentiment analysis
Text classification
Entity extraction
Modeling and tracking of discussed topics
GPT-like and ChatGPT-like models
By combining structured and unstructured data, organizations can comprehensively understand their operations and customer behavior, leading to more accurate and insightful analytics outcomes.
Data Governance and Security
Data governance and security are critical concerns when adopting AI-enabled analytics. Organizations must ensure compliance with data protection regulations and maintain the privacy and security of sensitive data. This issue becomes even more crucial as AI algorithms rely on vast amounts of data to make predictions and generate insights.
Here’s what you can do:
Organizations should establish clear policies and protocols for data handling, including data access controls, encryption mechanisms, and regular audits.
By implementing robust data governance frameworks and adhering to industry best practices, companies can maintain data integrity, safeguard customer information, and comply with regulations, building trust and confidence in their AI-enabled analytics capabilities.
Data quality and accessibility are formidable challenges faced by organizations embracing AI-enabled analytics. By implementing robust data governance practices, integrating disparate data sources, ensuring data quality, and prioritizing data security, companies can overcome these hurdles and unlock the full potential of AI-driven analytics. This transition will drive informed decision-making and gain a competitive edge in this data-driven era.
When you know how to navigate the data maze, it’s time to get started! That’s when you will see the power of AI-enabled data analytics.
Comments